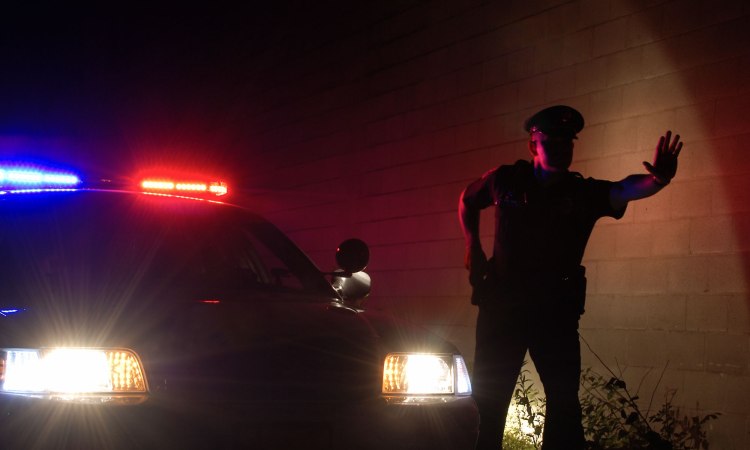
Justice should be blind, right? Unfortunately, the predictive policing software used in much of the US has bias and misunderstanding programmed right into it, says data scientist Cathy O’Neil.
The small city of Reading, Pennsylvania, has had a tough go of it in the postindustrial era. Nestled in the green hills 50 miles west of Philadelphia, Reading grew rich on railroads, steel, coal and textiles. But in recent decades, with all of those industries in steep decline, the city has languished. By 2011, it had the highest poverty rate in the country, at 41.3 percent. (The following year, it was surpassed, if barely, by Detroit.) As the recession pummeled Reading’s economy following the 2008 market crash, tax revenues fell, which led to a cut of 45 officers in the police department — despite persistent crime.
Reading police chief William Heim had to figure out how to get the same or better policing out of a smaller force. So in 2013 he invested in crime prediction software made by PredPol, a Big Data startup based in Santa Cruz, California. The program processed historical crime data and calculated, hour by hour, where crimes were most likely to occur. The Reading policemen could view the program’s conclusions as a series of squares, each one just the size of two football fields. If they spent more time patrolling these squares, there was a good chance they would discourage crime. Sure enough, a year later, Chief Heim announced that burglaries were down by 23 percent.
Predictive programs like PredPol are all the rage in budget-strapped police departments across America. New York City uses a similar program, called CompStat, and Philadelphia police are using a local product called HunchLab that includes risk terrain analysis, which incorporates certain features, such as ATMs or convenience stores, that might attract crimes. If you think about it, hot-spot predictors are similar to the shifting defensive models in baseball, a system that looks at the history of each player’s hits and then positions fielders where the ball is most likely to travel. Crime prediction software carries out a similar analysis, positioning cops where crimes appear most likely to occur. Both types of models optimize resources. But a number of the crime prediction models are more sophisticated, because they predict progressions that could lead to waves of crime. PredPol is based on seismic software: it looks at a crime in one area, incorporates it into historical patterns, and predicts when and where it might occur next. (One simple correlation it has found: If burglars hit your next-door neighbor’s house, batten down the hatches.)
Using predictive crime models creates a pernicious feedback loop — policing itself spawns new data, which justifies more policing.
Predictive crime models like PredPol have their virtues. Unlike the crime-stoppers in Steven Spielberg’s dystopian movie Minority Report, the cops don’t track down people before they commit crimes. Jeffrey Brantingham, the UCLA anthropology professor who founded PredPol, stressed to me that the model is blind to race and ethnicity. And PredPol doesn’t focus on the individual — instead, it targets geography. The key inputs are the type and location of each crime and when it occurred. If cops spend more time in the high-risk zones, foiling burglars and car thieves, there’s good reason to believe that the community benefits.
But most crimes aren’t as serious as burglary and grand theft auto, and that is where serious problems emerge. When police set up their PredPol system, they have a choice. They can focus exclusively on so-called Part 1 crimes (violent offenses that include homicide, arson and assault) or broaden their focus to include Part 2 crimes (which include vagrancy, aggressive panhandling and selling and consuming small quantities of drugs). Many of these “nuisance” crimes would go unrecorded if a cop weren’t there to see them, and these kinds of crimes are endemic to many impoverished neighborhoods.
Unfortunately, including nuisance crimes in the model threatens to skew the analysis. Once this data flows into a predictive model, more police are drawn into those neighborhoods, where they’re more likely to arrest more people. Even if their objective is to stop burglaries, murders and rape, they’ll have slow periods — it’s the nature of patrolling. And if a patrolling cop sees a couple of kids who look no older than 16 guzzling from a bottle in a brown bag, he stops them. These low-level crimes populate their models with more and more dots, and the models send the cops back to the same neighborhood.
This creates a pernicious feedback loop — the policing itself spawns new data, which justifies more policing. And our prisons fill up with hundreds of thousands of people found guilty of victimless crimes. Most of them come from impoverished neighborhoods, and most are black or Hispanic. So even if a model is color-blind, the result of it is anything but. In America’s largely segregated cities, geography is a highly effective proxy for race.
The police make choices about where they direct their attention — today they focus almost exclusively on the poor.
But what if police looked for crimes far removed from the boxes on the PredPol maps — the ones carried out by the rich? In the 2000s, the kings of finance threw themselves a lavish party. They lied, they bet billions against their own customers, they committed fraud and paid off rating agencies. The results of these crimes devastated the global economy for the best part of five years. Millions of people lost their homes, jobs and health care. And we have every reason to believe that more such crimes are occurring in finance right now.
Thanks largely to the industry’s wealth and powerful lobbies, finance is underpoliced. Just imagine if police enforced their zero-tolerance strategy in finance. They would arrest people for even the slightest infraction, whether it was chiseling investors on 401ks, providing misleading guidance or committing petty frauds. My point is that police make choices about where they direct their attention. Today they focus almost exclusively on the poor. That’s their heritage, and their mission, as they understand it.
Data scientists are stitching the status quo of the social order into models, like PredPol, that hold ever-greater sway over our lives. The result is that while PredPol delivers a perfectly useful and even high-minded software tool, it is also what I call a weapon of math destruction (WMD), or math-powered applications that encode human prejudice, misunderstanding and bias into their systems. PredPol, even with the best of intentions, empowers police departments to zero in on the poor, stopping more of them, arresting a portion of those, and sending a subgroup to prison. And the police chiefs, in many cases, if not most, think that they’re taking the only sensible route to combating crime. That’s where it is, they say, pointing to the highlighted ghetto on the map. And they have cutting-edge technology (powered by Big Data) reinforcing their position, while adding precision and “science” to the process. The result is that we criminalize poverty, believing all the while that our tools are not only scientific but fair.
The noxious effects of uneven policing that arise from predictive models do not end when the accused are arrested.
While looking at WMDs, we’re often faced with a choice between fairness and efficacy. American legal traditions lean strongly toward fairness. The Constitution, for example, presumes innocence and is engineered to value it. From a modeler’s perspective, the presumption of innocence is a constraint, and the result is that some guilty people go free, especially those who can afford good lawyers. Even those found guilty have the right to appeal their verdict, which chews up time and resources. So the system sacrifices enormous efficiencies for the promise of fairness. The Constitution’s implicit judgment is that freeing someone who may well have committed a crime, for lack of evidence, poses less of a danger to our society than jailing or executing an innocent person.
WMDs, by contrast, tend to favor efficiency. By their very nature, they feed on data that can be measured and counted. But fairness is squishy and hard to quantify — it is a concept. And computers, for all of their advances in language and logic, still struggle mightily with concepts, like beauty, friendship, and yes, fairness. Fairness isn’t calculated into WMDs, and the result is massive, industrial production of unfairness. If you think of a WMD as a factory, unfairness is the black stuff belching out of the smoke stacks. The question is whether we as a society are willing to sacrifice a bit of efficiency in the interest of fairness. Should we handicap the models, leaving certain data out? It’s possible, for example, that adding gigabytes of data about antisocial behavior might help PredPol predict the mapping coordinates for serious crimes. But this comes at the cost of a nasty feedback loop. So I’d argue that we should discard the data.
Besides fairness, another crucial part of justice is equality. That means, among many other things, experiencing criminal justice equally. Justice cannot just be something that one part of society inflicts upon the other. The noxious effects of uneven policing that arise from predictive models like PredPol do not end when the accused are arrested and booked in the criminal justice system. Once there, many of them confront the recidivism model used for sentencing guidelines. The biased data from uneven policing funnels right into this model. Judges then look to this supposedly scientific analysis, crystallized into a single risk score. And those who take this score seriously have reason to give longer sentences to prisoners who appear to pose a higher risk of committing other crimes. [Editor’s note: In late 2016, a devastating analysis by ProPublica showed how one such predictive algorithm, COMPAS, inaccurately identified black defendants as future criminals far more often than it named white defendants, and several scholars offered ways to tweak the algorithms to make them more fair.]
Sadly, in today’s age of Big Data, innocent people surrounded by criminals get treated badly, and criminals surrounded by a law-abiding public get a pass. And because of the strong correlation between poverty and reported crime, the poor continue to get caught up in these digital dragnets. The rest of us barely have to think about them.
Excerpted from the new book Weapons of Math Destruction: How Big Data Increases Inequality and Threatens Democracy by Cathy O’Neil. Reprinted with permission from Crown, an imprint of the Crown Publishing Group, a division of Penguin Random House LLC, New York. © 2016 Cathy O’Neil.