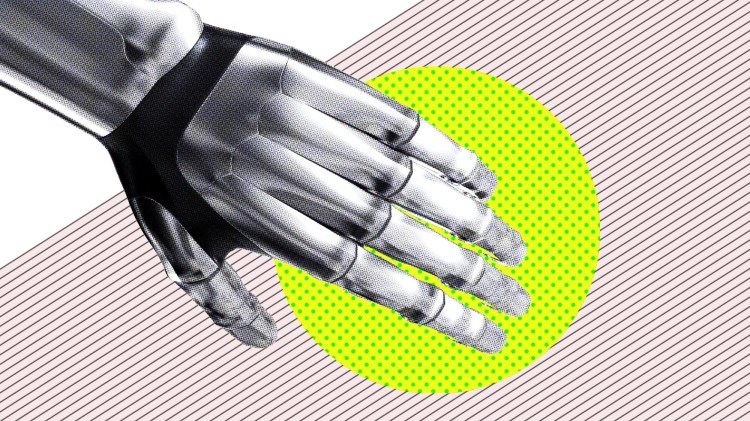
The race toward the Smart House of the future is barreling along, from Jibo the creepy home sidekick and Pepper the punny robot to near-military-grade vacuums. Yet between us and robotic domestic bliss, there remain some deceptively simple problems that take a large amount of computing.
Friction is very hard to account for.
To get a robot to pick up an object on its own, for example, is a challenge that has eluded roboticists for decades. Not only is there a danger of crushing the object, but friction is very hard to account for: Robots can’t calculate how easily something will slip out of their grasp, the way even a fourteen-month-old can. Then there’s figuring out what the object is and which way it’s meant to be oriented. Roboticist Rodney Brooks (TED Talk: Why we will rely on robots) lists three major challenges facing modern robotics: mobility, manipulation and messiness. Imagine you’re a robot, says Ken Goldberg of Berkeley (TED Talk: 4 lessons from robots about being human): “Everything is blurry and unsteady, low-resolution and jittery…. You can’t perfectly control your own hands. It’s like you’re wearing huge oven mitts and goggles smeared with Vaseline.”
To tackle the grasping problem, Goldberg’s team has turned to cloud robotics. His latest robots are programmed to draw on information stored in the cloud — and because they don’t need to carry burdensome amounts of processing power and the whole of known knowledge around with them, they can be faster and smarter, and can make rapid calculations on networked computers to perform complex tasks, like picking up an object of unknown weight, density and fragility.
To deal with so much uncertainty, the robot uses a type of statistical analysis called “belief space.”
Goldberg’s latest robot, for example, uses Google Goggles: Its built-in camera takes a photo of an object and sends that information to a database of images in the cloud to make an approximation as to what the object might be. Then a program associates its guess with another database of 3D models that suggests to the robot how it should pick up the object. To deal with so much uncertainty, the robot uses a type of statistical analysis called belief space. Built on partially observable Markov decision processes, or POMDPs, this analysis could require hundreds or even thousands of processors. Says Goldberg, “Until very recently it wasn’t feasible to do this, but with cloud computing, you can consider tens of thousands of perturbations of the object, its position, the grasper’s position, the shape — and you can integrate all those possibilities to figure out what action the robot could take that would have the maximum probability of success.”
Goldberg’s robot has an added feature: a system of Microsoft Kinect scanners that creates a new 3D model based on the object and stores it offline so other robots can learn from this initial interaction. In the future, says Goldberg, this robot-to-robot learning could happen in real time.
Should we be optimistic about robot impact on the lives of the elderly?
Goldberg thinks we’re going to see low-cost high-functioning robots in our homes in the next decade. He’s especially optimistic about the impact they’ll have on the lives of the elderly, which we’re going to have more of soon. Men and women over 65 will likely make up 20 percent of the American population by 2030 (up from 13 percent), and the number of caregivers per person over 80 will drop from 7.2 to 4.1. Robots that can grasp and pick up an object could be incredibly important. Says Goldberg: “For [a senior citizen] to slip and fall on something that was lying on the ground could be lethal. They could break a hip and then have all kinds of complications. There’s a lot of potential for a robot that would basically de-clutter homes.”
Goldberg’s robot fits into the larger narrative of the Internet of Things, a mix of pragmatic and absurd technologies that will someday pervade our homes. Says Goldberg, “You can imagine even later down the road: You buy a toy, and it has a built-in processor. So when a robot comes up to it and has never seen it before, essentially it asks the object, ‘Where do you belong? How should I pick you up?’ The object will say, ‘I’m heavier on the bottom side than on the left, and here’s a great place to pick me up.’ And then it can tell the robot, ‘And I belong with the preschool kids’ toys.’”
Featured artwork by Kelly Rakowski.