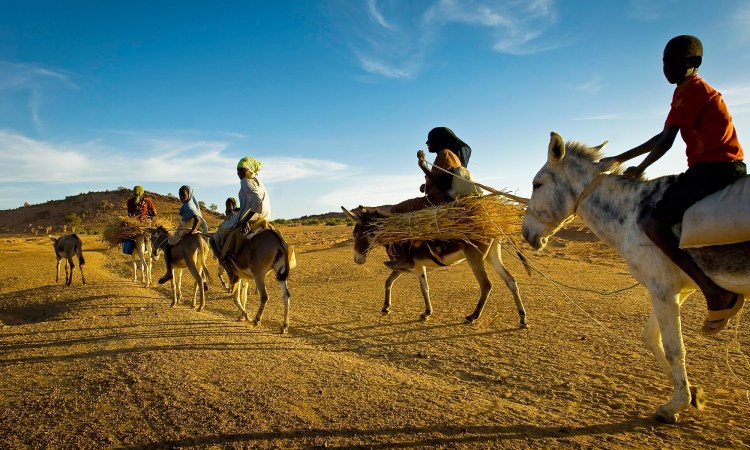
Refugee advocate Rana Novack is using AI to help predict new waves of migration — so the world can help before disaster strikes.
Mass migrations are part of our modern reality: The number of people forcibly displaced from their homes, by war or persecution, ballooned to 68.5 million people in 2017, according to the UN. Many of these people stay somewhere in their home countries, but a large group — some 25.4 million people by 2017 — have been forced to leave their countries altogether, officially becoming refugees. And “a reactionary response is just not the smartest way to deal with it,” says refugee advocate Rana Novack (TED@IBM Talk: How we’ll predict the next refugee crisis). That’s why she’s helping to develop a computational model in order to predict migrations between countries in a given year. If it works, the software could provide a potentially life-saving advantage to policy makers, humanitarian groups and national governments: the ability to plan ahead for a refugee crisis — before it happens.
In September 2015, Alan Kurdi, a three-year-old Syrian boy, became the symbol of the refugee crisis after a photo of his tiny body, lying dead on a Turkish beach, was published worldwide. For Novack, the tragic image epitomizes the failure of the international response to refugees. The Kurdi family had fled war-torn Syria for Turkey. Refugees couldn’t legally work there, and while the family hoped to immigrate to Canada, strict laws made it unlikely they’d be granted asylum in that nation. But without a safe or legal way to move somewhere they could work, they put their lives in the hands of human traffickers and tried to reach Greece in an inflatable boat. It capsized, and Alan and his five-year-old brother drowned, joining the over 3,600 other refugees who died while trying to cross the eastern Mediterranean in 2015. The world was shocked, but Novack wasn’t. “How could this come as a surprise?” she remembers thinking. “We didn’t leave them any other choice.”
Novack was familiar with this kind of desperation. The US-born daughter of Syrian immigrants, she was scrambling at the time to help her extended family in Syria relocate to a safe place. But when she called humanitarian organizations for advice, she was told point-blank: Her family members, like more than four million other Syrians in 2015, would have to cross the border on their own before they could get emergency assistance in a refugee camp. In other words, while the agencies knew that a massive crisis was unfolding in Syria, they had no plan; individuals and families had to make a potentially illegal, expensive, life-threatening border crossing before they could get any help. This made no sense to Novack. Working in business development at IBM, she knew about modern computing’s ability to predict future scenarios, even complex ones. “The idea of being able to predict a crisis, to proactively respond — it’s not rocket science,” she says. “Think of a hurricane or a flood. We can do these things for natural disasters, so why can’t we do these things for manmade disasters?”
Businesses already use sophisticated models to predict human behavior. “If they can tell me what kind of shirt I want to buy, there must be a way for us to predict the refugee crisis,” she recalls thinking. She began talking to the engineers around her. A team came together, including IBM researcher Rahul Nair, who was studying how people move within cities in order to design smarter bus networks. As Novack put it: “There’s a measurable set of circumstances. We can study them and we can analyze them. And we can better support these people.”
Many factors influence whether an individual will decide to flee their country, and where they’ll go if they do. Geographers call them “push-pull factors.” Push factors, such as unemployment, conflict and violence, are the ones that pressure people to leave their home countries. Pull factors, which include perceived economic opportunity or an existing immigrant community, might attract migrants to a particular nation. To capture these factors, the team compiled and parsed international news on migration going back to 2010, as well as development and economic data from sources like the World Bank going back to the 1960s. Another factor: the distance between countries, which is a quantity that encompasses more than simply mileage. As Nair points out, while migrants often try to stay closer at first — large numbers of Syrian refugees went to Lebanon, for example — migrants from Francophone Africa are drawn to France, whereas West Indians are likely to go to Britain, where strong communities exist because of past colonization. For each pair of linked countries, the team also fed in data representing physical distance, language and colonial links.
Computational models learn from past data to create a complex mathematical relationship between causes and effects. The process is known as supervised machine learning. The team gave its model the inputs — the historical data on these push, pull and distance factors for 189 countries — and the output — the last 15 years of migration numbers as sourced from the UNHCR. By analyzing these inputs and outputs, the model figured out a mathematical relationship between them so that, given push, pull and distance factors, it could calculate migration numbers for the year ahead. Just as a business algorithm might produce a long-range sales forecast, their strategic model predicts the average number of migrants between any two of 189 countries in the world over a coming year. Since many other forces can have a major impact on migration — such as when Hungary closed its border with Croatia in October 2015 — the team included the ability to add scenarios like a policy change or border closure to see how migration numbers are affected.
Once the team developed their model based on historical data, they tested it: Could it have predicted the 2015 European refugee crisis? When they input the push, pull and distance factors that were known at the time, the model then calculated refugee flows in 2015. When the team checked these numbers against real data for 2015, the average error rate was about 1,000 people per year per country. This means the model doesn’t work for small migrations, says Nair — for example, the number of Irish people going to Australia. But in mass migrations, such as the 5.6 million people who have left Syria since 2011, the model could be accurate enough to help make decisions.
The team also created a version of its model that produces short-term forecasts, so NGOs can plan for refugee movements in the very near future. Like a short-term weather forecast, this “operations model” takes recent refugee arrival data, news and current weather for a given area, and then projects arrivals at refugee camps on a day-by-day basis, up to three weeks out. A “flow model” predicts the number of people in a given refugee camp who are expected to move to another camp — in the same country or a different one — over this period. These kinds of models could help organizations make better decisions about moving resources and staff among the refugee camps they serve.
This data-based approach to migration gave new insights that weren’t being captured on the ground. Typically,NGOs can only record the number of people arriving at a camp on a given day. By looking at the larger picture, the IBM team determined the rate at which people were moving between camps, and how long it took for them to travel, say, from a refugee camp in Greece to one in Austria. They saw new trends as well: for instance, high wind speeds on the Mediterranean correlated strongly with fewer arrivals in Greece. It makes sense, Nair says: “Smugglers and facilitators along the route would not launch their boats on windy days.”
The IBM team is now collaborating with humanitarian organizations in Europe to fine-tune their software. They’ve started a project with the Danish Refugee Council (DRC), which is surveying Ethiopian refugees on their way out of the country about why they leave. This data could help improve the IBM model’s ability to predict the movement of refugees, because migration trends sometimes run counter to what you might expect, according to Nair. For example, two countries could have the same GDP, but many more people might flee from one nation than the other. “There’s not a simple formulaic prescription for why people would leave,” he says, and the Ethiopian survey data will allow the team to start to tease out this complexity. While it’s still too early to report results, the team has started combing through the DRC survey data with the goal of mapping personal motives to the existing data on economic and conflict-related factors. That could help them understand which pressures are more important than others, and how exactly these larger forces interact to make a person leave. A more detailed picture of the complex dynamics of migration should result in a truer-to-life model and a sharper ability to predict when and how mass movements may occur rather than being caught by surprise by a flood of people. In the future, Nair also dreams of bringing in other sources of data, such as their mobile phone location. “Migrants are particularly sensitive to having phones; they keep them charged on journeys because they know that this at least gives them a contact,” he says. The phones, like all mobile devices, constantly share their locations with nearby cell towers; that, in turn, could provide finer-grained information about refugee flows. But of course, says Nair, the team would have to figure out how to do this while respecting privacy laws.
The goal is to predict the next refugee crisis before it happens. Since the team’s strategic forecast provides refugee movements over a coming year (the furthest ahead they can currently predict with any degree of accuracy), it’s technically possible to set up safe ways for people to leave their countries before they’re desperate enough to turn to smugglers and human traffickers, like Alan Kurdi’s family did. “I think of the people putting their kids in a raft, and every story I’ve read about people dying in the back of a truck … it was because they were relying on a human trafficker to get them from point A to point B,” Novack says. “If we knew the pathways that people were taking ahead of time, then we could reinforce those pathways” and save lives. She dreams of policy makers making proactive, positive decisions about refugees at the national level. For example, two months after Kurdi’s death in September 2015, Canada revised its immigration policy to bring in 10,000 Syrian refugees by the end of that year; it has since welcomed tens of thousands more. But Novack says, “Why do we we wait years into a crisis when thousands of people have drowned, hundreds of thousands are killed, millions are displaced, and only then we talk about opening our doors?”
Another good question: How can tech firms create more technology like this, tech for the greater good, not just for profit? Novack’s team is part of IBM’s philanthropic arm, but most humanitarian organizations can’t afford to develop sophisticated software like this. As Novack puts it, “There’s an innovation gap in the humanitarian sector.” She calls for tech companies to use their expertise to help. Quite simply, as she puts it in her talk, “we have to make sure that the people who want to do the right thing have the tools and the information they need to succeed.”